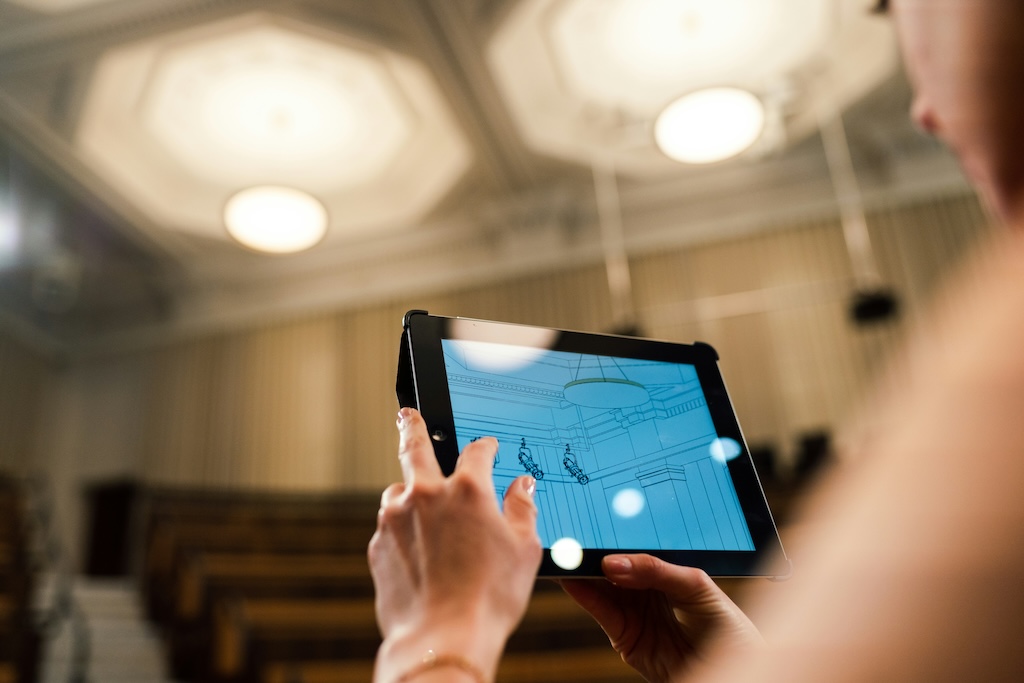
Getting Started with Data Analytics and AI Applications
Artificial Intelligence (AI) is revolutionizing how businesses operate, offering transformative potential across various domains. AI data analytics, AI predictive analytics, and AI applications are at the forefront of this revolution, enabling organizations to harness vast amounts of data to drive innovation, efficiency, and growth.
This guide will help you understand how to start and scale your journey toward AI maturity, exploring key AI capabilities, foundations of data analytics, and practical steps to build an effective AI portfolio.
What is AI Data Analytics?
AI data analytics refers to the application of AI technologies to analyze large datasets and extract actionable insights. Unlike traditional analytics, AI analytics utilizes machine learning algorithms to identify patterns, trends, and anomalies within data, providing deeper and more accurate insights.
Understanding Data Requirements
The first step is to identify the data required for your specific AI use case. This involves determining the relevant data sources, the volume of data needed, and the level of data quality and completeness necessary for accurate model training and inference.
Data Preprocessing and Cleaning
Raw data is often noisy, inconsistent, and incomplete. Data preprocessing techniques like handling missing values, removing duplicates, and formatting data ensure that the dataset is clean and ready for analysis. This step is critical for improving data quality and model performance.
Exploratory Data Analysis
Exploratory data analysis (EDA) involves visualizing and summarizing the key characteristics of the data. EDA helps identify patterns, outliers, and relationships between variables, providing valuable insights for feature selection and model development.
Feature Engineering
Feature engineering is the process of creating new features from the existing data that better represent the underlying problem to the algorithms. This may involve transforming variables, creating interaction terms, or extracting advanced features using techniques like natural language processing or computer vision.
Model Selection and Evaluation
Based on the problem and data characteristics, appropriate machine learning models are selected and evaluated. This involves splitting the data into training and testing sets, training multiple models, and assessing their performance using relevant metrics like accuracy, precision, recall, or mean squared error.
Data Analytics Tools and Platforms
While data analysis requires significant domain expertise, there are many data analytics tools and platforms available that can accelerate the process. These data analytics solutions provide user-friendly interfaces, automated pipelines, and advanced algorithms to streamline tasks like data ingestion, wrangling, visualization, and modeling. By leveraging data analytics technologies, organizations can democratize access to insights, empower citizen data analysts, and drive more informed decision-making across teams. Integrating data analytics capabilities with AI initiatives enables a smooth translation of analytical findings into deployed AI models and applications.
Ensuring Data Security and Privacy
As organizations increasingly rely on data for AI machine learning initiatives, protecting data and privacy becomes paramount. Robust data governance policies and protocols must be established to protect sensitive information and maintain regulatory compliance. This includes implementing access controls, data encryption, anonymization techniques, and stringent auditing processes.
Driving AI Value Through People, Process, and Systems
To unlock the full potential of artificial intelligence, it's crucial to focus on three pillars: people, processes, and systems. Consider a framework for communicating your organization’s current state and how it can mature in AI capabilities. Establish clear metrics to measure AI performance and align outcomes with organizational goals. Custodial control ensures that AI actions align with intended outcomes, while continuous coaching and feedback foster ongoing improvement.
Data scientists must deeply understand the tasks they're automating, and management should communicate the project's goals effectively to ensure buy-in and engagement. By refining processes over time based on data from each digital and physical process, organizations can build a roadmap for continuous AI-driven innovation.
People
Effective communication ensures the success of AI initiatives. Management must articulate the project's goals clearly, fostering buy-in and engagement across all levels of the organization. When employees understand the purpose behind AI integration and are actively involved in its implementation, they can become champions of change rather than passive bystanders. Look for ways to embed data-driven approaches and technology-forward thinking into your team with easy AI wins like enhancing business intelligence & reporting.
Organizations must foster an environment where data scientists deeply understand the tasks they're automating. By bridging the gap between AI capabilities and domain expertise, data scientists can develop solutions that not only automate processes but also enhance them, driving efficiency and innovation.
Process
Implementing AI isn’t a one-and-done endeavor; it's an ongoing process of refinement. Organizations must establish clear metrics to measure AI performance and align outcomes with strategic objectives. Custodial control ensures that AI actions remain aligned with intended outcomes, preventing unintended consequences.
Continuous coaching and feedback mechanisms are also essential. By collecting data from both digital and physical processes and soliciting feedback from operators to leadership, organizations can drive continuous improvement. With this mindset of ongoing improvement, organizations can build a roadmap for AI-driven innovation, constantly evolving and adapting to meet changing business needs.
Systems
The success of AI initiatives hinges on the robustness of the underlying systems. To support AI deployment effectively, organizations must invest in the technological infrastructure, organizational processes, and culture that support artificial intelligence integration.
Achieving Strategic Objectives with AI
The true value of AI lies not in its technological capabilities alone but in its ability to empower organizations to achieve their strategic objectives. By focusing on the pillars of people, processes, and systems, organizations can unlock the full potential of AI, driving innovation, efficiency, and value creation across the enterprise.
Building Your AI Portfolio: Essential Steps
With a sound foundation of people, process, and systems, it’s time to envision and build your artificial intelligence portfolio. But building an AI portfolio requires planning and consideration to bring the right pieces together to enable success. As you begin building your AI portfolio, let’s consider how Data Availability, People, and Implementation Speed play unique roles.
Assessing Data Availability
At the heart of any successful AI initiative lies high-quality data. Before diving headfirst into AI implementation, take stock of your data assets. Ensure they are clean, reliable, and relevant to your business objectives. If your data is lacking in any aspect, invest in data collection, cleaning, and enrichment processes. This is a key step early in AI maturity that enables immense value.
Identifying Key People for AI Initiatives
AI is not just about technology—it's also about people. Identify individuals within your organization who are not only proficient in AI technologies but are also open to change. These change champions will play a crucial role in driving AI adoption across various departments. Encourage a culture of continuous learning and provide training opportunities to upskill employees in AI-related competencies. By fostering a team of AI enthusiasts, you'll pave the way for smoother integration and widespread acceptance of AI initiatives.
Ensuring Rapid Implementation
In today's competitive landscape, time is of the essence. When selecting artificial intelligence solutions for your portfolio, prioritize those that offer rapid implementation without compromising on quality. Companies mature in AI analyze data, make sense of it, and seek proven solutions to problems in their organization.
Look for solutions that seamlessly integrate with your existing systems, minimizing disruption to day-to-day operations. Consider AI platforms that come with pre-built models and connectors, enabling you to hit the ground running. Many AI applications are tailored to specific business functions, such as AP automation, order entry optimization, or inventory management. These off-the-shelf solutions not only accelerate time-to-value but also reduce implementation risks. By capitalizing on existing AI frameworks, you can focus your resources on customization and optimization, rather than starting from square one.
Putting It All Together
Building an AI portfolio is a strategic endeavor that requires careful planning and execution. By prioritizing data quality, nurturing a culture of innovation, embracing rapid implementation, and leveraging pre-built solutions, you'll set your company on the path to AI-driven success. Remember, the AI landscape is constantly evolving, so stay agile, stay informed, and stay ahead of the curve. Consider systems that already have all the pipes connected. This is where buying can help accelerate value. Consider AI for AP automation, AI for order entry, and AI for inventory optimization through TORI. With the right mindset and approach, the possibilities of AI are limitless.
Three Things Artificial Intelligence Does Well
As companies begin and progress in their journey of embracing data analytics, automation, and more with AI tools, understanding the distinct strengths of artificial intelligence becomes increasingly vital. Understanding these strengths illuminates the potential AI use cases across the organization. Let’s look at three key capabilities of AI and their significance for organizations maturing in their data and AI utilization: prescriptive analytics, sense and respond, and generative AI.
Prescriptive Analytics
One of AI's most remarkable abilities lies in its capacity to handle vast amounts of data and derive meaningful insights from it. Through prescriptive analytics, AI algorithms can sift through complex datasets, identify patterns, and predict future outcomes with remarkable accuracy. This capability empowers businesses to make informed decisions, optimize processes, and drive innovation.
Example: AI for Supply Chain Networks
A supply chain network can be modeled by analyzing freight costs, warehouse costs, and sourcing locations to determine the optimal distribution centers for delivering and receiving goods as well as enable you to examine the impacts of various network changes. This can have immense benefits including lower freight costs, reduced warehousing costs, minimized mileage, and data-driven decision-making for supply chain networks.
Sense and Respond
AI's ability to interpret and respond to real-time data streams has revolutionized industries ranging from transportation to healthcare. Whether it's analyzing video feeds to detect anomalies or processing sensor data to identify potential equipment failures, artificial intelligence enables proactive decision-making in dynamic environments.
Example: AI for Manufacturing Automation
Consider the application of AI capabilities in manufacturing automation. Data is being collected through machines and IoT sensors constantly. This data can be utilized to build models and automation that configure machines and run manufacturing processes.
Generative AI
Perhaps one of the most intriguing aspects of AI is its ability to generate content, engage in natural language interactions, and automate repetitive tasks. Generative AI algorithms can create realistic images, compose music, and even write compelling stories—all without human intervention.
Example: AI for Marketing
Imagine a marketing team utilizing artificial intelligence to generate personalized email campaigns tailored to individual customer preferences. By analyzing past interactions and demographic data, AI can craft engaging content that resonates with each recipient, driving higher engagement and conversion rates.
AI Tools for the Mundane and Complex
In addition to content creation, generative AI holds immense potential in automating mundane tasks, freeing up valuable time for employees to focus on more strategic initiatives. Whether it's generating code snippets, drafting legal documents, or composing music, AI-powered automation streamlines workflows and boosts productivity across various domains.
Artificial intelligence offers capabilities that empower businesses to innovate, optimize processes, and stay ahead of the competition. From prescriptive analytics to sense and response capabilities to generative AI, the potential applications of AI are vast and diverse.
Navigating the Future of AI
The future of AI is here, and it's multi-modal. Imagine a world where your operations are not just efficient but anticipatory, where downtime is minimized, and productivity soars to new heights. That's the promise of Generative AI, leading the charge toward more sophisticated and versatile applications across industries. From manufacturing to healthcare, finance to transportation, the opportunities are boundless. Companies must ensure that they are growing in their AI maturity by solidifying fundamentals and being prepared to embrace new technology as it develops.
Potential Artificial Intelligence Projects
The path to AI maturity is a journey, not a destination. As organizations progress along this path, they will encounter a wide array of potential AI projects and use cases. These projects can span different domains, functional areas, and levels of complexity, enabling companies to incrementally build their AI capabilities and drive value across the enterprise:
Sample projects across different levels of AI maturity include:
- Copilot integration for enhanced productivity
- AI-powered accounts payable automation
- Accelerated order entry processes
- Back-office automation for streamlined operations
- Early warning systems for operational decisions
- Demand forecasting
- Price optimization
- Inventory optimization
- Production scheduling
- Truck routing
- Supply chain network design
- Industry-specific solutions to gain a competitive edge
Industry-Specific Benefits of Artificial Intelligence
What could this look like in different industries? In manufacturing, for example, Generative AI enables the integration of data from various sources—sensor data, visual inspections, and historical maintenance records—to predict equipment failures with unprecedented accuracy. This proactive maintenance approach minimizes downtime and optimizes production efficiency, giving you a competitive edge in a rapidly evolving market.
But the potential of AI extends far beyond manufacturing. In every sector, AI is reshaping the way we work and interact with technology. Whether it's enhancing patient care in healthcare, driving smarter investment decisions in finance, optimizing logistics in transportation, or defining the right pricing strategy, AI is revolutionizing operations and opening doors to new possibilities.
A Culture of Continuous Improvement
The future of artificial intelligence isn't just about adopting new technology, it's about fostering a culture of continuous improvement. At AMEND, we're committed to partnering with you every step of the way, from strategy development to implementation and beyond. Together, we'll cultivate an environment that encourages innovation, embraces change, and prioritizes learning and adaptability.
Embracing Innovation Today for Success Tomorrow
Achieving AI maturity involves understanding the foundations of successfully scaling with artificial intelligence, building a robust AI portfolio, and exploring industry-specific use cases. By focusing on data availability, key personnel, and rapid implementation, businesses can unlock the full potential of AI.
Next Steps for AI Implementation
Organizations should start with small AI projects, gradually scaling up as they build expertise and confidence. By continuously evaluating AI initiatives against strategic goals, you can ensure alignment and ongoing value.
Where do you stand on the Maturity Scale?
Whether you have a defined problem or are looking for an AI use case, the AMEND team is ready to partner with you on your journey to full AI maturity.
Schedule a call today to unlock the power of AI for your business tomorrow.