The AMEND Process
Design and Discovery
Met extensively with the executive team to fully understand the strategic goals of the business. This discovery uncovered the need to better assess member health and capture drivers of churn.
Data Architecture
In collaboration with the IT team, we connected and cleaned data sources into a single source of truth in Power BI.
Model Development and Implementation
Using more than 15 years of member churn data, a logistic regression model was built to predict the likelihood of churn by member and report back on the five biggest drivers of churn.
The Solution
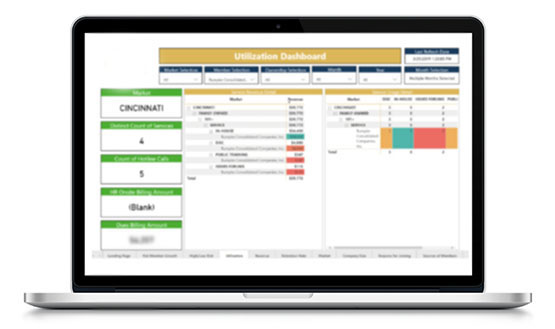
Churn Probability Model
We built an algorithm that leverages usage, interaction and sales data by member and product type to predict the likelihood of churn for each member, each year.
This model uncovers what matters, sets expected member and dollar losses annually and identifies members at risk of churn.

Power BI Dashboard
We developed a reporting mechanism to highlight at-risk customers and the drivers of that risk.
This dashboard is utilized by several departments in the organization including client services, sales, finance, and marketing to target retention opportunities, determine retention strategies, standardize practices, and set budgets.
Results
Planning
Set appropriate net member growth goals
Metrics
Effectively tested retention metrics and identified retention risk
Budget
Implemented a budgeting strategy for new target development